We all know machine translation is pretty bad, but what about machine
transcription? After trying to seriously transcribe some lectures in an online course I’m nominally taking,
1 I have to say: Pretty bad too.
The most serious problem that machine transcription suffers from is one that machine translation also suffers from: sometimes it will transcribe something that means exactly the
opposite of what is being said. It can also skip words, get confused by stutters, and synthesize words that are not there in the audio (and that you will have a hard time coming up with an explanation of why they have been put there).
So let’s look at something “interesting”:
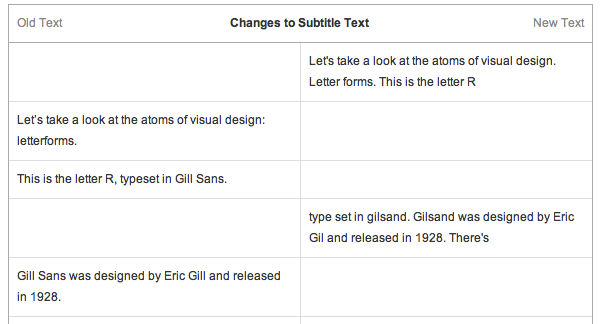
The left shows part of the transcript of a lecture I transcribed, and on the right is the corresponding machine transcription. What immediately stands out is that it got the personal name wrong (sometimes it does get names right, sometimes even admirably well); and then
—
if you are familiar with graphic design or printing
—
you will notice that it missed the words “letterforms” and “typeset”.
There are, of course, the problems of poor punctuation and strange formatting. But let’s move on, further in the same transcript:
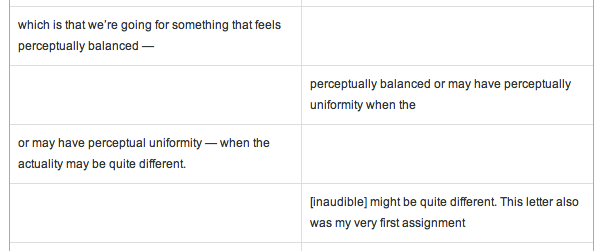
Here we have the word “actuality” in the audio that I could fairly easily make out but that the machine could not. Sometimes it’s the opposite (especially when the audio is practically completely garbled), but a lot of times you are just amazed at how it fails to transcribe words that are crystal clear in the audio.
Yet further along in the same transcript:
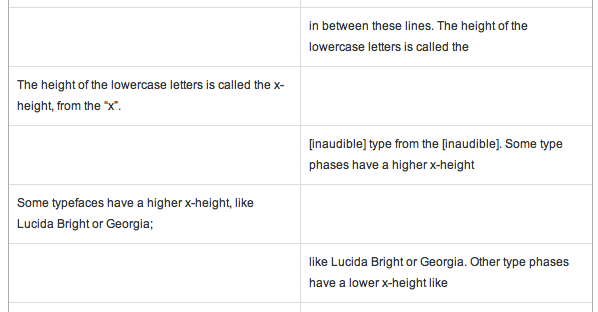
Here, the speech recognition failed to transcribe the words “x-height” and “typefaces”. While you could argue that machines are not experts in graphic design or printing, this still makes you wonder how it could have transcribed the word “type” in there. And in any case it transcribed two other instances of the word “x-height” correctly, so it really is a mystery why it failed to transcribe the first one.
Audio is not always easy to transcribe, especially when the person speaks too fast or too softly, or murmurs, or has an unusual accent. This course’s lecturer does not really have an accent, but he does say the words “ascender” in a very strange way (perhaps that is the way they pronounce it at
RISD), which totally confused the machine:
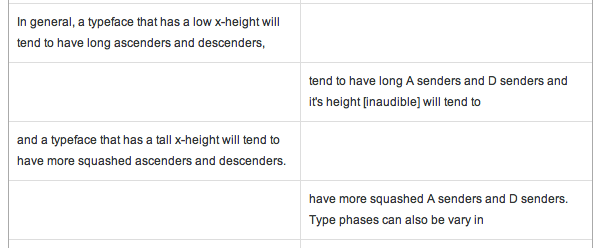
If someone had no prior typographic knowledge and the transcript is all they got, there is no way they could figure out what “A senders” and “D senders” are. In a couple of lectures he spoke unusually softer than normal, and that completely threw the speech recognizer off.
The machine also completed missed the words “and a typeface that has a tall”. I don’t have more screen shots of how it misses half a sentence or generates half a sentence of phantom words, but this could be the next best:
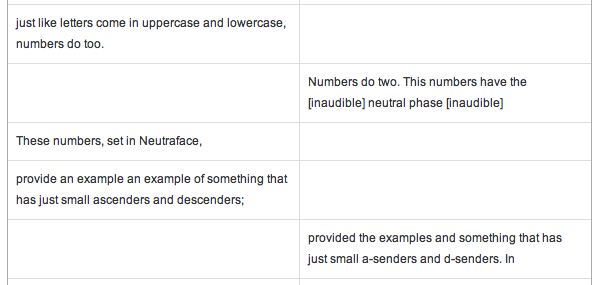
I did make a transcription mistake (“an example” transcribed twice), but the speech recognizer basically screwed up the whole thing. Practically close to half of the words were incorrectly transcribed.
As I mentioned before, sometimes machines are really good at making out personal names but sometimes not:
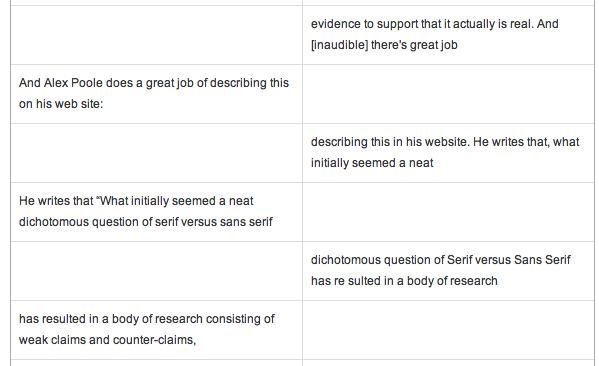
It also spelt “serif” and “sans serif” as if they were real font names, and inserted a space into the word “resulted”. The latter, of course
—
to people who have seen
PDF-to-text output often enough
—
, is the result of having the speech recognizer trained on
PDF files.
Honestly, I really doubt if machine-generated transcripts like this are really useful for hard-of-hearing students. And these inaccurate transcripts
—
which form the basis for translations
—
are outright detrimental to students who will be learning from translated subtitles.
If you have finished a proper transcript and then the machine came along and replaced your work with something inferior like this, will you do nothing? No way.
Notes:
1 Coursera’s lectures are transcribed by a speech recognition system operating under the name of “stanford-bot”, and in a sense you may say that the identity of this system has been veiled in secrecy
—
even the teaching staff do not seem to know about its existence. In a couple of courses (SaaS and
NLP) there were debates on whether the initial subtitles were created through speech recognition or by human volunteers.
One described the transcripts as “fantastically high precision” if it was a machine;
others saw glaring errors. The question was finally settled in June when I finally contacted them to complain about the “user”, and the replies I got confirmed my suspicious: that “user” is actually a machine. [
Back to text]